Tommy Berger Eriksen is a Senior Health Specialist in Skretting Aquaculture Innovation where he works in our biotechnology centre, The Bubble. He specialises in histology, the study of tissue using microscopy.
How AI revolutionises digital microscopy of fish and shrimp tissues at Skretting
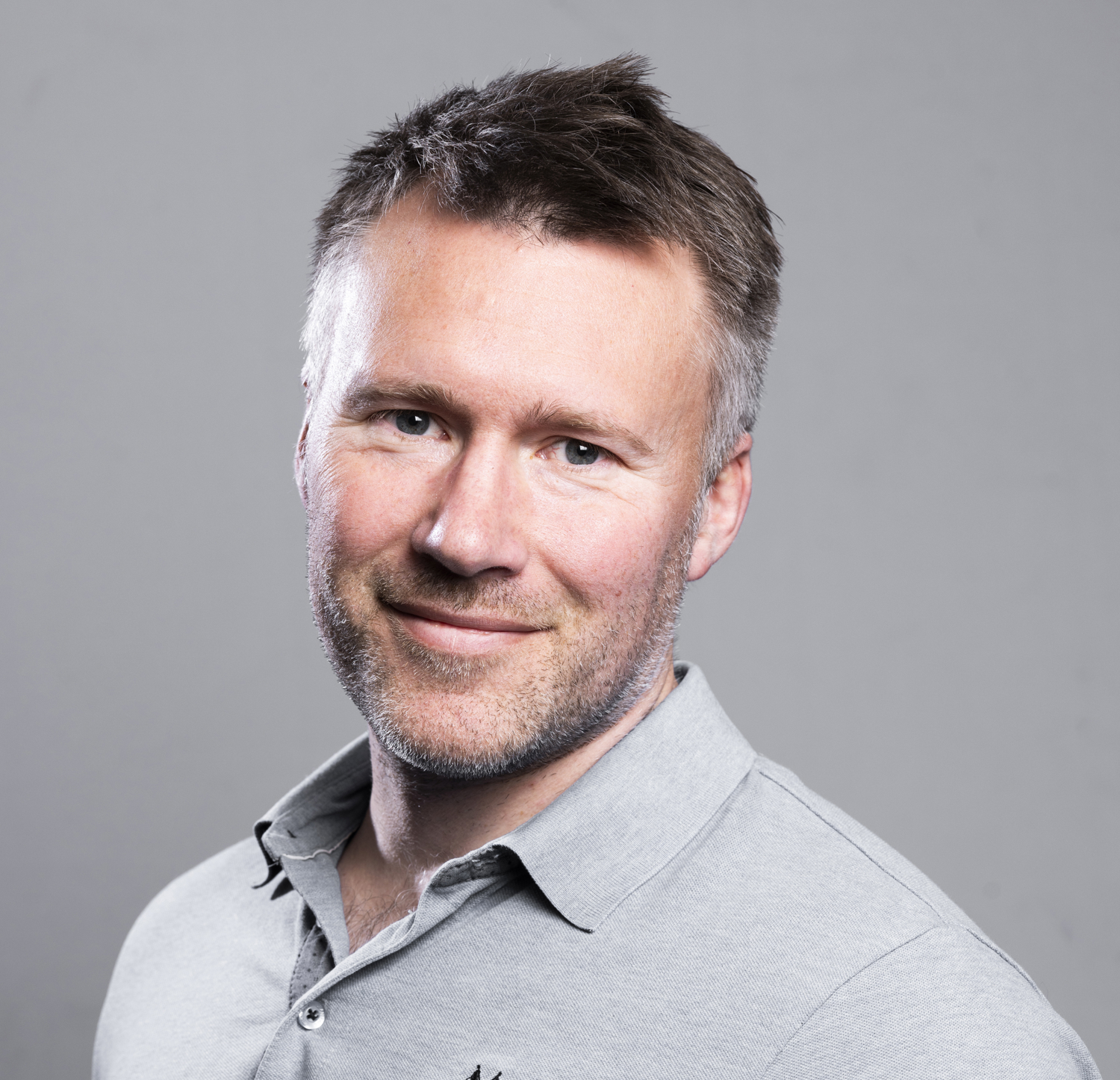
Histology is a gold standard method for assessing human and animal health . At Skretting, we have been optimising this microscope-based method for the last two decades, with multiple PhD projects and collaborations with world-class institutions such as universities and hospitals. In The Bubble, we have state of the art equipment including a high-throughput microscope slide scanner that can convert up to 150 microscope slides at a time into incredibly high resolution virtual slides that reveal such astonishing details that we can even zoom in on individual cells. We aspire to work with the best, are at the forefront of advancing scientific methods in the realm of digital histology, and are pioneering its use in fish and shrimp health.
Since Tommy started working with histology in 2011, his role has always involved multiple processes including planning and coordination, staining tissues, digitalisation, image analysis and interpreting results. Now, with AI, things are being taken to the next level.
"The part where tissues are classified in the digital images, and pixels are sorted into different categories, is the most difficult and time-consuming part. And since 2021, we’ve been using AI to assist with this”, explains Tommy.
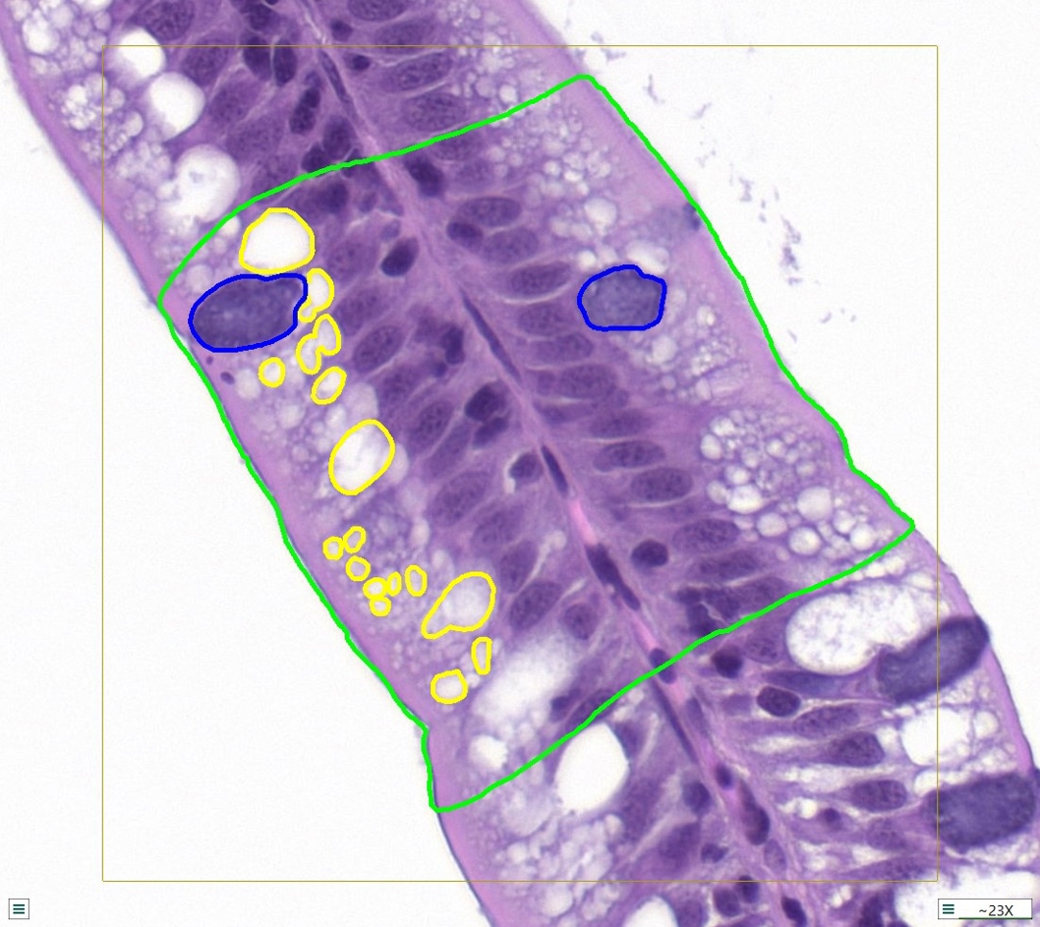
Show it: The operator annotates/draws examples on the images to be shown to the AI.
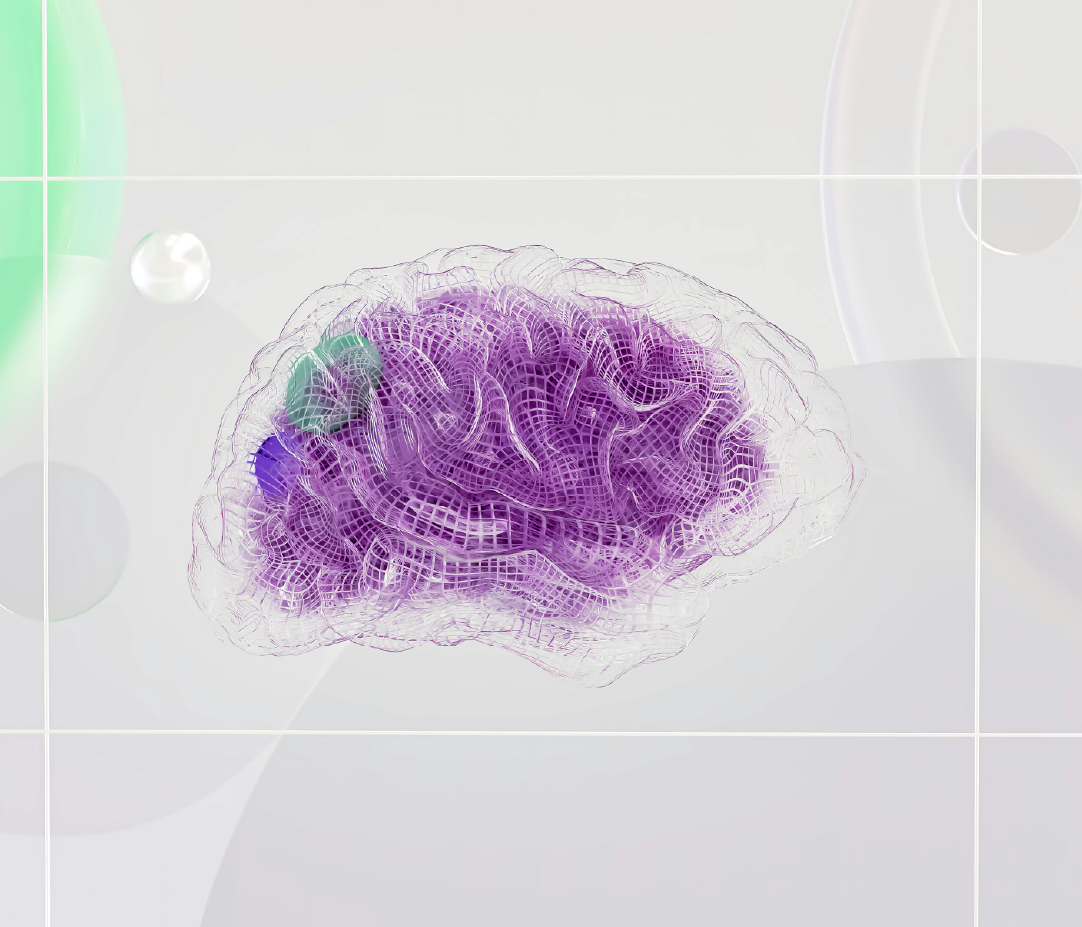
Train it: The AI is presented with the annotated examples. The AI uses the images to train itself on how to analyse the tissues in accordance with the examples provided. Then, it produces an algorithm.
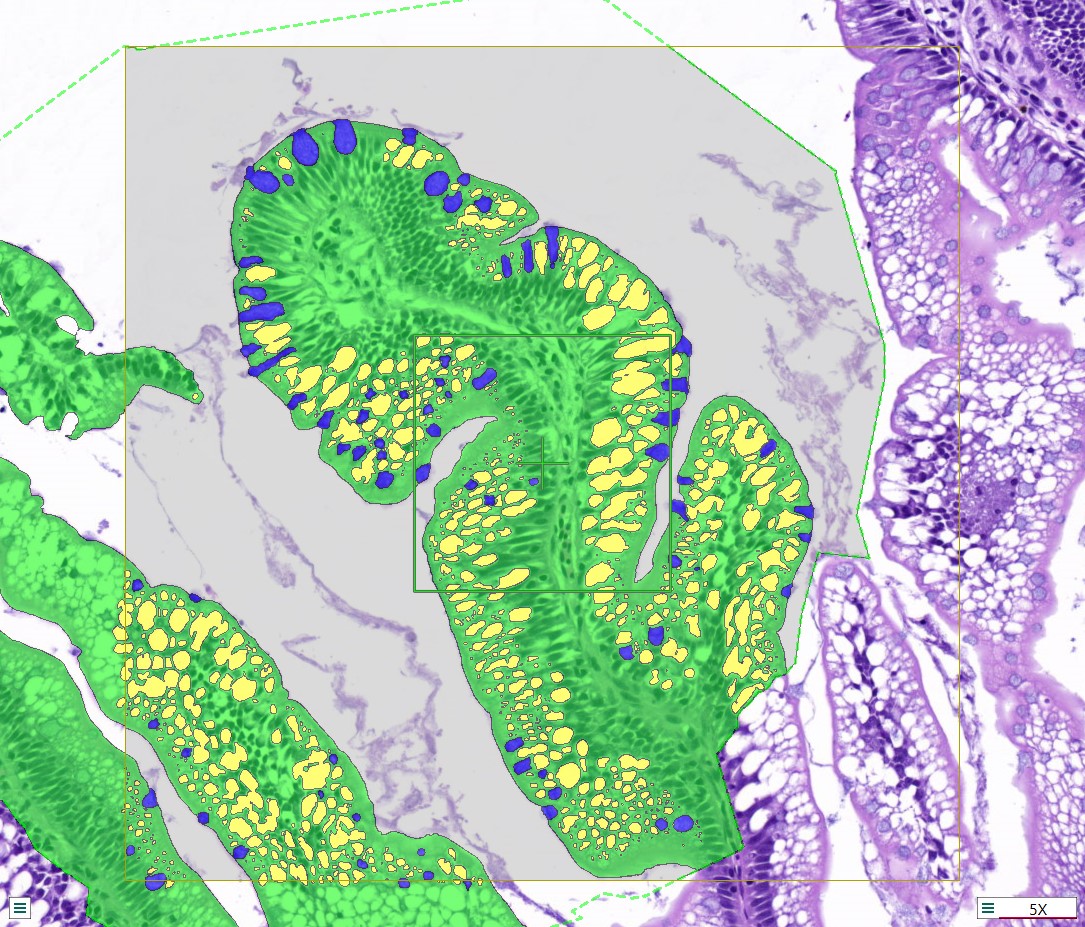
Find it: Now, the operator can provide numerous images to the AI and it will automatically find the data requested.
Training the AI to classify specific cells or regions of interest requires identifying a wide variety of examples. “This is a crucial step”, says Tommy. “The goal is to develop an AI that can analyse multiple projects, even when there are variations in tissue structure or staining color. For example, if one project’s samples have a much darker staining color than usual, the AI might misinterpret the data unless provided with similar examples during training.”
Once examples are identified, Tommy meticulously annotates the different cells and regions of interest, a process that can take days or even weeks, before feeding the data to the AI for training. “Depending on the number of examples and factors such as complexity, hardware, and image resolution, the training process can take anywhere from a few hours to several days. The AI continually refines its algorithm by comparing its classifications to the provided annotations. “We aim for the AI’s classifications to match our annotations with 98-99% accuracy. Even though my annotations might not be perfect for every single cell, the AI is smart enough to deduce the correct patterns based on the average quality of my annotations.”
Tommy’s work training the AI has demanded patience and precision, but the results speak for themselves. Today, the AI can isolate a region of interest such as the epidermis from 400 skin samples in under an hour—a task that would take a pathologist 40 hours to complete manually. “And since it’s automated, you can spend that hour doing something else,” Tommy points out. While developing robust AI takes time, the payoff is substantial, saving a tremendous number of man-hours.
“My work isn’t done, though,” he laughs. “We’ve made great progress in annotating and classifying salmon tissues, particularly for the intestine, skin, and gills. But there’s still ongoing work with other organs and species, like shrimp. There’s always more to do—refining algorithms, feeding the AI more examples, and improving accuracy.”